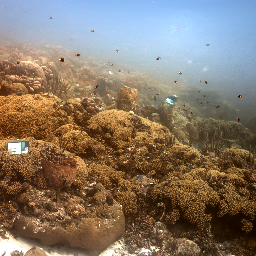
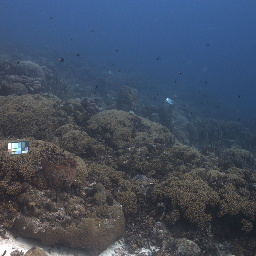
Underwater image restoration is a challenging task because of water effects that increase dramatically with distance.
This is worsened by lack of ground truth data of clean scenes without water.
Diffusion priors have emerged as strong image restoration priors.
However, they are often trained with a dataset of the desired restored output, which is not available in our case.
We also observe that using only color data is insufficient, and therefore augment the prior with a depth channel.
We train an unconditional diffusion model prior on the joint space of color and depth, using standard RGBD datasets of natural outdoor scenes in air.
Using this prior together with a novel guidance method based on the underwater
image formation model, we generate posterior samples of clean images, removing the water effects.
Even though our prior did not see any underwater images during training, our method outperforms state-of-the-art baselines for image restoration on very challenging scenes.
Our code, models and data are available on the project’s website.
Comparison with several underwater methods.
Select a scene from the upper row and a method from the right column.
The input underwater image is labeled '1. Underwater,' and our result is '8. Osmosis'.
The Osmosis result is shown on the left side, with the compared method displayed on the right.
Comparison with several underwater monocular depth methods.
Select a scene from the upper row and a method from the right column.
The input underwater image is labeled '1. Underwater,' and our result is '7. Osmosis'.
The Osmosis result is shown on the left side, with the compared method displayed on the right.
The iterative sampling process starts in \(t = T\) with random noise in 4 channels. The denoising step outputs denoised samples \(\hat{x}_0=\hat{J}_0, \hat{D}_0\). We use the physical image formation model together with \(\hat{x}_0\) to optimize the water parameters \(\phi\), and to guide the sampling towards the observed image. This process repeats itself, gradually updating both the estimated image and depth, until \(t = 0\), in which \(\hat{x}_0\) hold the method’s estimate for the reconstructed scene \(\hat{J}_0\) and its depth \(\hat{D}_0\)
In water, we observe two wavelength- and distance- dependent effects. First, the \(\textit{direct}\) signal reflected from the object is attenuated. Second, light is scattered onto the object's line-of-sight (LOS), creating an additive signal termed \(\textit{backscatter}\) that increases with distance. The occluding backscatter layer is independent of the scene content. Thus, the visibility and contrast of further objects is significantly reduced and their colors are distorted.
@article{nathan2024osmosis,
title={Osmosis: RGBD Diffusion Prior for Underwater Image Restoration},
author={Bar Nathan, Opher and Levy, Deborah and Treibitz, Tali and Rosenbaum, Dan},
journal={arXiv preprint arXiv:2403.14837},
year={2024}
}